Ranter
Join devRant
Do all the things like
++ or -- rants, post your own rants, comment on others' rants and build your customized dev avatar
Sign Up
Pipeless API
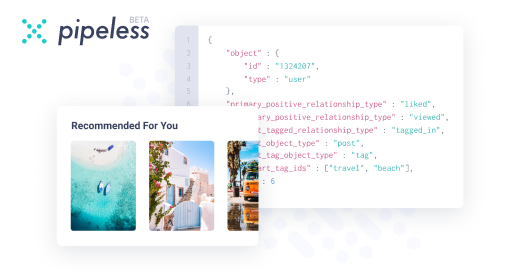
From the creators of devRant, Pipeless lets you power real-time personalized recommendations and activity feeds using a simple API
Learn More
Comments
-
typosaurus11005230d@SidTheITGuy would love to but the model is currently very dead. I'm rewriting the connection from my home server to my vps server. I have to convert everything to websocket communication instead of the default protocol that it has. My model is a python project, not native model anymore so it can't work on the original protocol. Stupid that they don't support websocket out of the box. They do provide a http endpoint that can stream, but it's sessionless or not async... I've tried a 136Mb AI model. I was suprised! It was called smollm2. The one i'll be using is prolly 8gb or so
-
Wisecrack9274207dInstead of training the networks, just instance the graph multiple times into several variations, and select subsets of weights and biases from each instance. Randomly adjust or reinitialize those subsets, then do a few minibatches and measure the MLE of each instance and merge the most accurate one (for some flavor of 'accurate') back to the parent graph.
No backprop needed in training.
I'm trying to find a variation of this that works for forward passes during inference, but I haven't yet.
Hypothetically this should work better than even AdamW because a sufficiently random source should always approximate the gaussian distribution for a given training regime way better than any amount of training and validation data you can get your hands on.
No local minima that can't be overcome!
Related Rants
My model has learnt the most important stuff about devRant first. Including its biggest mystery. See screenshot (image upload still broken) https://devrant.molodetz.nl/2024-11....
It drives me crazy, will we ever find out?
Some people say he's on a German Opel/Vauxhall forum now having fun with vroemtards instead of us. I do find myself in a jealous position. "Ngl" like Sid would say. Don't judge, many 30+ year old speak gen Z.
Also, I've heard he drives daily to work in a Lada from an communist era. He has to because he went from self employed back to a loan slave and his new boss refused to pay his expensive public transport tickets while being late every time.
But one thing is almost certain at the moment! He's gonna marry!
(Seriously dude, if she knows your internet history and she still loves you, never let her go)
random
ostream
we probably don't know it
love
the bot probably knows it
marriage