Join devRant
Do all the things like
++ or -- rants, post your own rants, comment on others' rants and build your customized dev avatar
Sign Up
Pipeless API
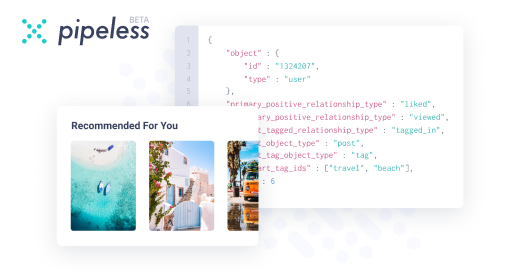
From the creators of devRant, Pipeless lets you power real-time personalized recommendations and activity feeds using a simple API
Learn More
Search - "overfitting"
-
Dropout layers improve neural net learning by randomly "killing neurons" thus preventing overfitting.
That's how I will justify my alcoholism from now on.7 -
Adaptive Latent Hypersurfaces
The idea is rather than adjusting embedding latents, we learn a model that takes
the context tokens as input, and generates an efficient adapter or transform of the latents,
so when the latents are grabbed for that same input, they produce outputs with much lower perplexity and loss.
This can be trained autoregressively.
This is similar in some respects to hypernetworks, but applied to embeddings.
The thinking is we shouldn't change latents directly, because any given vector will general be orthogonal to any other, and changing the latents introduces variance for some subset of other inputs over some distribution that is partially or fully out-of-distribution to the current training and verification data sets, thus ultimately leading to a plateau in loss-drop.
Therefore, by autoregressively taking an input, and learning a model that produces a transform on the latents of a token dictionary, we can avoid this ossification of global minima, by finding hypersurfaces that adapt the embeddings, rather than changing them directly.
The result is a network that essentially acts a a compressor of all relevant use cases, without leading to overfitting on in-distribution data and underfitting on out-of-distribution data.13 -
One of my minions (erm, I mean, "a valued junior member of my team") asked to be assigned to tasks more "data science related".
Regardless of the very last-decade sounding request, I tried to explain to the Jr that there is more to "data science" than distilling custom llms and downloading pytorch models. There are several entire fields of study. And those are all sciences. In this context, science equals math.
But they said they were not scared of math.
I've seen them using their phones to calculate freaking tips. If you can't do 15% of a lunch bill in your head, hypothesis tests might be a bit more than challenging.
But, ok then. Here we go.
So I had them do some semi-supervisioned clustering. On a database as raw as dirt, but with barely 5Gb, few dimensions and regarding subjects with easily available experts.
Even better, we had hundreds of manually classified training and test cases.
The Jr came back a month later with some convoluted mess of convoluted networks; just the serialized weights of the poor thing were about as large as the database itself.
And when I tried it on some other manually classified test datasets... Freaking 41% error rate, for something that should be a slam dunk. Little better than a coin toss.
One month of their time wasted on an overfitted unusable mess.
I had to re-assign the task to someone else, more experienced, last friday. It was monday when they came up with an iterative KNN approach giving error rates for several values of K... some of them with less than 15% error on the test dataset.
WTF are schools teaching and calling "data science" nowadays?!?!?
I reeeeally need to watch those juniors more closely. Maybe ask for middle-sprint demonstrations. But those are soooo boring and waste so much time from people who know what they are doing...
Does anyone have a better idea to prevent this type of off-track deviation? Without being a total bore, that is.
And... should I start asking people "gotcha" data analysis questions before giving them free reign on this type of tasks? Or is it an asshole boss move? I would hate someone giving me a pop quizz before letting me work... But I got no other ideas.3 -
Some business users have been chasing me all week to produce a report using some old report with some modifications.
I didn't write the old code and have no context as to what the data is.
My current reaction is:
so you want a report that says X using some vague input which you haven't clearly defined or explained to me...
Have you heard about black boxes and overfitting (i.e. reverse engineering a process based on sample data)?
TLDR: I can generate a report that will say anything you want it to say... doesn't mean it will be right in future use cases.
Why don't people (originally GBoard suggested peepee) understand "junk in = junk out" -
Turns out you can treat a a function mapping parameters to outputs as a product that acts as a *scaling* of continuous inputs to outputs, and that this sits somewhere between neural nets and regression trees.
Well thats what I did, and the MAE (or error) of this works out to about ~0.5%, half a percentage point. Did training and a little validation, but the training set is only 2.5k samples, so it may just be overfitting.
The idea is you have X, y, and z.
z is your parameters. And for every row in y, you have an entry in z. You then try to find a set of z such that the product, multiplied by the value of yi, yields the corresponding value at Xi.
Naturally I gave it the ridiculous name of a 'zcombiner'.
Well, fucking turns out, this beautiful bastard of a paper just dropped in my lap, and its been around since 2020:
https://mimuw.edu.pl/~bojan/papers/...
which does the exact god damn thing.
I mean they did't realize it applies to ML, but its the same fucking math I did.
z is the monoid that finds some identity that creates an isomorphism between all the elements of all the rows of y, and all the elements of all the indexes of X.
And I just got to say it feels good. -
friend: yo dude, wanna drink?
me: nah man, that stuff kills brain cells.
friend: you say its killing brain cells but i say its just real life dropout to prevent overfitting1 -
analogy for overfitting :
cramming a math problem by heart even the digits of any problem for exam.
now if the exact same problem comes to exam i pass with full marks else if just the digits are changed however the concept is same and simce i mugged up it all rather than understanding it i fail. -
Here's one for the data scientists and ML Engineers.
Someone set a literal date feature (not month, not season, but date) as a categorical feature... as a string type 🥺
I don't trust this model will perform for long2 -
js developer during the day, python developer at night. The constant switch is almost impossible to adapt to and I see myself up to 2 times per functiin/merhod wondering why this block won't run, just to realize, that implemented a feature from the other language. IDEs provide much less support for script languages than typestrict languages. The choice of libraries is nicely overfitting all your needs and most of the documentation reminds me of my teachers, because they also like to simply force their logic on you, without explaining the backgrounds.
Script languages are fun2 -
I'm beginning to feel like any kind of specific approximation via neural networks is a myth. That if you can't reduce output to simple categorical values that can be broadly interpreted between two points, that it doesn't work.
I have some questions and they don't seem to be getting answered about the design of the net. How many layers should I use ? How many neurons per layer ? How does this relate to the number of desired quantitive scalar outputs I'm looking to create, even if they are normalized, they can vary GREATLY and will if I'm approximating the out of several mathematical expressions. Based on this and the expected error ranges of these numbers and how many possible major digits could be produced within the domain of the variable inputs being introduced, how many neurons per layer ? What does having more layers do ? In pytorch there don't seem to be a lot of layer types per say, but there are a crap ton of activation functions, and should I just be using these at the tail end or should they actually be inserted between layers so the input of the next layer passes through another series of actiavtion functions ? what does this do to the range of output ?
do I need to be a mathematician to do this ?
remembered successes removed quantifiable scalars entirely from output, meaning that I could interpret successful results from ranges of decimal points.
but i've had no success with actual multi variable regression as of yet, even when those input variables are only 2 and on limited value ranges eg [0,100] and [0, 2pi]
and then there are training epochs to avoid overfitting, and reasonable expectation of batches till quality results will start to form.3