Join devRant
Do all the things like
++ or -- rants, post your own rants, comment on others' rants and build your customized dev avatar
Sign Up
Pipeless API
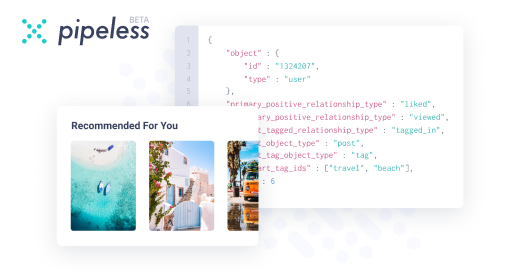
From the creators of devRant, Pipeless lets you power real-time personalized recommendations and activity feeds using a simple API
Learn More
Search - "performance tuning"
-
To me, it seems like the rise of distributed systems like mesos / kubernetes combined with Docker require you to be master sysadmin, veteran kernel hacker and a part time c developer ALL AT ONCE if you really want to shave off time from debugging/ performance tuning sessions. Anyone wish they paid more attention in class ? Lol.4
-
Client: *uses Oracle on AWS RDS*
Ora: *licence about to expire*
Client: we need a cheaper and equally performant solution.
AWS: we have an outstanding Aurora db! Its performance is flawless!
Client: shut_up_and_take_my_money.png
Engineers: *migrating to Aurora mysql*
Engineers: fine-tuning the db for 2 months, adapting app code.
Aurora: *shits its pants during test ramp-up, delivers ~70% of Ora perf*
==========
Client: we need to do better!
AWS: try Aurora Postgresql!
Engineers: *migrating to Aurora postgresql*
Engineers: fine-tuning the db for 1 month.
Aurora: *shits its pants during test ramp-up, delivers ~40% of Ora perf*
AWS: let me see...
AWS DBAs: *fine-tuning the db for another 2 months*
Aurora: *survives ramp-up, delivers ~70% Ora perf*
AWS DBAs: your application is wrong.
We: Ora didn't complain about that...
AWS DBAs: umm.. Err.. Then your load test is wrong
We: ora wasn't complaining about that either...
AWS DBAs: errr.. Ummm.. Oh, I've got it! Your queries aren't optimized!
We: we cannot change queried - they are hardcoded by our framework's vendor
AWS DBAs: well there you go! Your queries aren't optimized! It's your fault, not aurora's
yyeeahhhh... Riight...
From my xp, aws support and aws engineers ALWAYS try to put the blame on a client. Always. Even when they're obviously wrong.15 -
I'm having an existential crisis with this client.
We are spending millions of $s every year to make sure the product's performance is perfect. We are testing various scenarios, fine-tuning PLABs: the environment, application, middleware, infra,... And then we provide our recommendations to the client: "To handle load of XX parallel users focusing on YY, yy and Zy APIs, use <THIS> configuration".
And what the client does?
- take our recommendations and measure the wind speed outside
- if speed is <20m/s and milk hasn't gone bad yet, add 2x more instances of API X
- otherwise add 3xX, 1xY and give more CPUs to Z
- split the setup in half and deploy in 2 completely separate load-balanced prod environments.
- <do other "tweaking">
- bomb our team with questions "why do we have slow RTs?", "why did the env crash?", "why do we have all those errors?", "why has this been overlooked in PLABs?!?"
If you're improvising despite our recommendations, wtf are we doing here???
One day I will crack. Hopefully, not sometime soon.3 -
The next step for improving large language models (if not diffusion) is hot-encoding.
The idea is pretty straightforward:
Generate many prompts, or take many prompts as a training and validation set. Do partial inference, and find the intersection of best overall performance with least computation.
Then save the state of the network during partial inference, and use that for all subsequent inferences. Sort of like LoRa, but for inference, instead of fine-tuning.
Inference, after-all, is what matters. And there has to be some subset of prompt-based initializations of a network, that perform, regardless of the prompt, (generally) as well as a full inference step.
Likewise with diffusion, there likely exists some priors (based on the training data) that speed up reconstruction or lower the network loss, allowing us to substitute a 'snapshot' that has the correct distribution, without necessarily performing a full generation.
Another idea I had was 'semantic centering' instead of regional image labelling. The idea is to find some patch of an object within an image, and ask, for all such patches that belong to an object, what best describes the object? if it were a dog, what patch of the image is "most dog-like" etc. I could see it as being much closer to how the human brain quickly identifies objects by short-cuts. The size of such patches could be adjusted to minimize the cross-entropy of classification relative to the tested size of each patch (pixel-sized patches for example might lead to too high a training loss). Of course it might allow us to do a scattershot 'at a glance' type lookup of potential image contents, even if you get multiple categories for a single pixel, it greatly narrows the total span of categories you need to do subsequent searches for.
In other news I'm starting a new ML blackbook for various ideas. Old one is mostly outdated now, and I think I scanned it (and since buried it somewhere amongst my ten thousand other files like a digital hoarder) and lost it.
I have some other 'low-hanging fruit' type ideas for improving existing and emerging models but I'll save those for another time.6 -
By implementing proper eager loading in Eloquent, based on a sample request, I reduced it from 850 DB queries down to just 14 DB queries. So that's an increase in 98% performance?
-
My team has a Database Admin 2 position open on the Arvest Career site. We are looking for someone with Data Warehousing/Data Integration background with SQL Server, ETL, SSIS, or equivalent. Also looking for a physical DBA with background in SQL Server, performance tuning, partitioning, DR/HA, Database migrations, dB refresh, dB restore, building out clusters.
https://appone.com/MainInfoReq.asp/... -
Teaching coworkers performance tuning, we have the memory enough that you don't need to write to disk... Really the data isn't Even a MB....